
Young Scientist Lecture 3:00-4:00 PM June 14 (GMT+8:00)
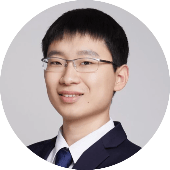
Speaker: Yuntian Chen
Title: An adaptive deep-learning load forecastingframework by integrating Transformer and domain knowledge
Speaker bio: Yuntian Chen is anassistant professor (Ph.D. Supervisor) at EIAS. His research field includes scientific machine learning and intelligent energy system. He is interested in the integration of domain knowledge and data-driven models. He graduated from the Department of Energy and Power Engineering of Tsinghua University with a dual bachelor's degree in economics from Peking University. He obtained Ph.D. degree from Peking University with merit. He was the co-founder of RealAI. He is a member of the Young Editorial Board of Advances in Applied Energy.
Abatract: Electrical energy isessential in today's society. Accurate electrical load forecasting is beneficial for better scheduling of electricity generation and saving electrical energy. In this talk, we will discuss an adaptive deep-learning load forecasting framework by integrating Transformer and domain knowledge (Adaptive-TgDLF). Adaptive-TgDLF introduces the deep-learning model Transformer and adaptive learning methods (including transfer learning for different locations and online learning for different time periods), which captures the long-term dependency of the load series, and is more appropriate for realistic scenarios with scarce samples and variable data distributions. Adaptive learning can cope with the change of load in location and time, and can make full use of load data at different locations and times to train a more efficient model. We also preliminarily mine the interpretability of Transformer in Adaptive-TgDLF, which may provide future potential for better theory guidance.
Time and date :
11:00-12:00 PM June 13 (GMT-8:00)
3:00-4:00 PM June 14 (GMT+8:00)
Zoom details:
The Zoom link is: https://shorturl.at/bhzEF
The Password is: 016185
Supporting institution:
Eastern Institute forAdvanced Study (EIAS)
Paper link:
https://www.sciencedirect.com/science/article/pii/S2666792423000215