
YOUNG SCIENTIST LECTURE
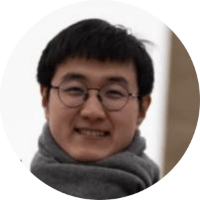
Speaker: MR. Ning Zhao
Title: New York State's 100% renewable electricity transition planning under uncertainty using a data-driven multistage adaptive robust optimization approach with machine-learning
Speaker bio : Ning Zhao is currently a Ph.D. student in Prof. Fengqi You’s group at Cornell University. His current research focuses on energy systems decarbonization planning using optimization and machine learning tools. His previous research projects include incentive energy policy design, decarbonization planning under uncertainty, and multi-scale renewable energy transition.
Supporting institution: Cornell University
ADAPEN paper link: https://doi.org/10.1016/j.adapen.2021.100019
Abstract: Power system decarbonization is critical for combating climate change, and handling systems uncertainties is essential for designing robust renewable transition pathways. In this study, a bottom-up data-driven multistage adaptive robust optimization (MARO) framework is proposed to address the power systems’ renewable transition under uncertainty. To illustrate the applicability of the proposed framework, a case study for New York State is presented. Machine learning techniques, including a variational algorithm for Dirichlet process mixture model, principal component analysis, and kernel density estimation, are applied for constructing data-driven uncertainty sets, which are integrated into the proposed MARO framework to systematically handle uncertainty. The results show that the total renewable electricity transition costs under uncertainty are 21%-42% higher than deterministic planning, and the costs under the data-driven uncertainty sets are 2%-17% lower than the conventional uncertainty sets. By 2035, on-land wind and offshore wind would be the major power source for the deterministic planning case and robust optimization cases, respectively.